We will continue to make mistakes in digital health and AI innovation, but if we invest in generating evidence earlier and more often, we can accelerate adoption of safe and effective digital interventions.
You don’t have to look far to see the results of not iteratively generating evidence for digital health and AI–in fact, you can just look at my research career! But before I share my small but painful lesson, let’s look at a big example of failure: the well meaning Australian government.
We would never consider developing a drug, asking a few associates to try it out and let us know their thoughts, then begin using it regularly in our hospital. But we are quick to implement AI solutions without much evidence. Similar to the translational research pathway for drug development, we need a pathway for generating evidence throughout the lifecycle of the digital intervention, matching the type of evidence to the stage of development. Although there is no single evidence generation pathway for all digital interventions, I agree with a white paper by Roche:
A key feature of an evidence generation pathway is rapid iteration in the early stages to guide refinement before moving forward to a more defined product that requires more rigorous assessment.
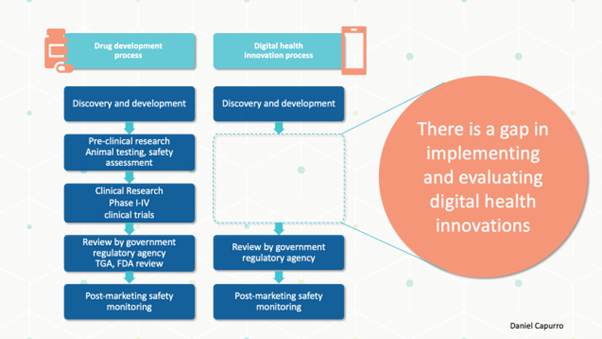
Jeremy Knibbs, publisher of our sister publication Health Services Daily, told a cautionary tale about an Australian government website called Medical Costs Finder to help consumers find and understand costs for GP and medical specialist services across Australia. On its face, this seems really useful–knowing expected out-of-pocket costs for a knee replacement, for example, can contribute to decision making, help prepare for costs, and
provide a guide for comparison against an actual quote from a provider. However, after spending $23 million, very few consumers use or even know about the site, and the estimates are based on only 52 specialists out of a potential of over 40,000 that could have signed up.
From what was reported, Medical Costs Finder is a compelling example of the need for iterative evidence generation with the willingness to modify or even abandon the plan based on answers to questions like these:
- Are you solving a real problem?
- Will people use the proposed solution?
- What is the best way to implement the solution?
- Does the solution provide value?
- Will the solution scale?
Knibbs concludes:
Australia needs to be very careful to prove its use cases, for providers and patients, and then be very sure (test a lot early) that whatever they intend to build will end up being useful to providers and patients in a manner that will get them to engage at scale with whatever is built.
Under my guidance, my research lab spent many years developing a tool called Knowledge Author to help people without natural language processing (NLP) expertise develop a knowledge base to drive NLP algorithms.
As I look back at the paper, I realise I still love this project! We published a journal article demonstrating that it performed well. But it joined the thousands of other papers and tools in the “valley of death” between an innovation and its routine use.
I relied on personal experience and anecdotes to answer the questions above and didn’t test the assumptions throughout development. If I had done some small studies along the way to answer those questions, I may not have developed what I set out to build, but I would have either pivoted to something more needed or modified the development toward something that had a greater chance of being adopted.
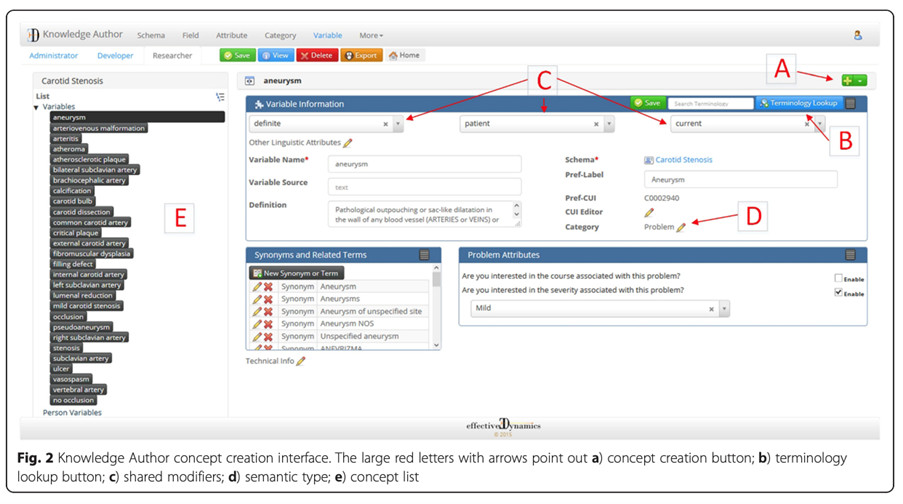
Luckily, I’m not alone in this experience, and people are sharing roadmaps, frameworks, strategies, bootcamps, and infrastructure to support frequent and iterative evidence generation from idea to prototype to silent pilot to implementation to monitoring and scaling.
Related
At the Centre for Digital Transformation of Health we are building a pathway to not only provide a list of the types of questions you should answer at different stages but also access to multidisciplinary expertise, digital tools, and realistic clinical and home space to assist innovators in generating that evidence efficiently.
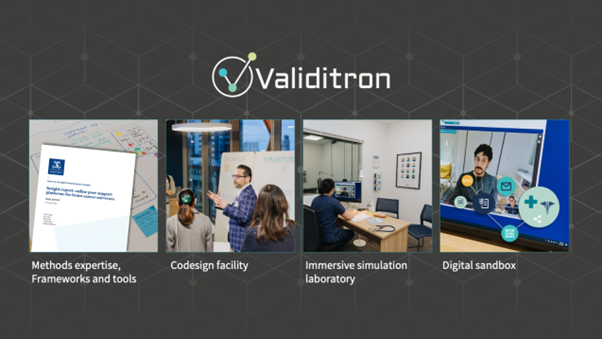
We will continue to make mistakes in digital health and AI innovation, but if we invest in generating evidence earlier and more often, we can correct course and accelerate adoption of safe and effective digital interventions. The next big question is:
Who is going to pay for the infrastructure and rigour needed to ensure safe and effective implementation of AI and other digital interventions?
Professor Wendy Chapman is a biomedical informaticist. She is director of the University of Melbourne’s Centre for Digital Transformation of Health.
This article was first published on Professor Chapman’s website. You can read the original here.